Reality AI Lab is pushing the boundaries of artificial intelligence, focusing on applications that directly impact our daily lives. We’re exploring how AI can solve real-world problems, from improving healthcare to enhancing environmental sustainability. This isn’t just theoretical research; we’re building practical solutions and exploring the ethical implications along the way. Our work spans several key areas, from developing cutting-edge AI models to establishing robust data management systems.
The lab’s core mission is to responsibly advance AI research and development, ensuring that these powerful technologies benefit society as a whole. We are committed to transparency and collaboration, working with experts across multiple disciplines to achieve our goals. This involves rigorous testing, ethical considerations, and a commitment to long-term sustainability.
Reality AI Lab: A Deep Dive
The Reality AI Lab is a hypothetical research facility dedicated to pushing the boundaries of artificial intelligence in the realm of real-world applications. Its mission is to develop and deploy cutting-edge AI solutions that address critical challenges across various sectors, while adhering to the highest ethical standards. This exploration will delve into the lab’s core functionalities, research areas, technological underpinnings, and potential societal impact.
Core Mission and Goals
The Reality AI Lab’s primary goal is to bridge the gap between theoretical AI advancements and practical, real-world deployments. This involves developing robust, reliable, and ethical AI systems capable of interacting with and interpreting complex real-world data. Key objectives include fostering innovation in AI research, creating tangible societal benefits through AI applications, and training the next generation of AI researchers and practitioners.
Potential Applications of Research
The research conducted within the Reality AI Lab has the potential to revolutionize numerous sectors. Applications span diverse fields, including:
- Healthcare: Developing AI-powered diagnostic tools, personalized medicine, and robotic surgery assistance.
- Environmental Science: Creating AI models for climate change prediction, resource management, and pollution monitoring.
- Smart Cities: Designing intelligent transportation systems, optimizing energy consumption, and improving public safety.
- Manufacturing: Automating production processes, enhancing quality control, and predicting equipment failures.
Technological Infrastructure
Supporting a state-of-the-art Reality AI Lab requires a robust technological infrastructure. This includes:
- High-performance computing clusters for training complex AI models.
- Large-scale data storage and management systems to handle massive datasets.
- Advanced networking capabilities for efficient data transfer and collaboration.
- Specialized hardware, such as GPUs and TPUs, optimized for AI computations.
- Secure data centers to protect sensitive information.
Research Areas within Reality AI Lab
The Reality AI Lab focuses on three key research areas: Computer Vision, Natural Language Processing (NLP), and Reinforcement Learning. Each area presents unique challenges and opportunities.
Computer Vision Research
This area focuses on enabling computers to “see” and interpret images and videos. Potential breakthroughs include real-time object detection with high accuracy, robust 3D scene understanding, and AI-powered image generation with unprecedented realism. Challenges include handling noisy data, ensuring fairness and bias mitigation in algorithms, and managing the computational demands of processing high-resolution images and videos. Opportunities lie in applications like autonomous vehicles, medical imaging analysis, and advanced robotics.
Natural Language Processing Research
This area centers on enabling computers to understand, interpret, and generate human language. Potential breakthroughs include truly conversational AI agents, accurate machine translation across multiple languages, and advanced sentiment analysis. Challenges involve handling ambiguity, context, and diverse language styles. Opportunities exist in improving customer service, automating language translation, and creating more accessible information for individuals with disabilities.
Reinforcement Learning Research
This area focuses on developing AI agents that learn through trial and error. Potential breakthroughs include creating AI agents capable of complex decision-making in dynamic environments, optimizing resource allocation in complex systems, and designing more adaptive and robust AI systems. Challenges include dealing with reward sparsity, ensuring safety and stability of learned behaviors, and developing interpretable reinforcement learning models.
Opportunities exist in robotics, game playing, and resource management.
AI Models and Algorithms Used in Reality AI Lab
The Reality AI Lab employs a variety of AI models and algorithms tailored to specific research tasks. A hypothetical workflow is Artikeld below.
Examples of AI Models
The lab utilizes models like Convolutional Neural Networks (CNNs) for image processing, Recurrent Neural Networks (RNNs) and Transformers for NLP, and various reinforcement learning algorithms such as Q-learning and Deep Q-Networks (DQNs). CNNs excel at image recognition but can struggle with abstract concepts. RNNs are adept at sequential data but can be computationally expensive. Transformers offer superior performance in NLP tasks but require significant computational resources.
DQNs are effective for learning complex actions but can be difficult to train and interpret.
Hypothetical Model Workflow
- Data Collection and Preparation: Gathering and cleaning relevant datasets.
- Model Selection: Choosing the most appropriate AI model based on the research task.
- Model Training: Training the selected model using the prepared dataset.
- Model Evaluation: Assessing the model’s performance using appropriate metrics.
- Model Deployment: Deploying the trained model into a real-world application or system.
- Model Monitoring and Refinement: Continuously monitoring the model’s performance and making necessary adjustments.
Ethical Considerations
The Reality AI Lab prioritizes ethical AI development. This involves careful consideration of bias in datasets, ensuring fairness and transparency in algorithms, and mitigating potential risks associated with AI deployment. Regular ethical reviews are conducted to ensure alignment with responsible AI practices.
Data Acquisition and Management in Reality AI Lab
The Reality AI Lab utilizes diverse methods for data acquisition and employs robust data management strategies to ensure data quality, security, and privacy.
Data Acquisition and Processing
Data acquisition involves collecting data from various sources, including sensors, databases, and publicly available datasets. Data preprocessing involves cleaning, transforming, and formatting the data to be suitable for AI model training. This may include techniques like data augmentation, normalization, and feature engineering.
Reality AI Lab is pushing the boundaries of artificial intelligence, exploring cutting-edge applications. Sometimes, though, you need a quick check to see if your tools are working; if you’re wondering “is chatgpt down?”, check this helpful site: is chatgpt down. Knowing the status of these platforms is crucial for seamless integration into projects at Reality AI Lab, ensuring our research runs smoothly.
Data Security and Privacy
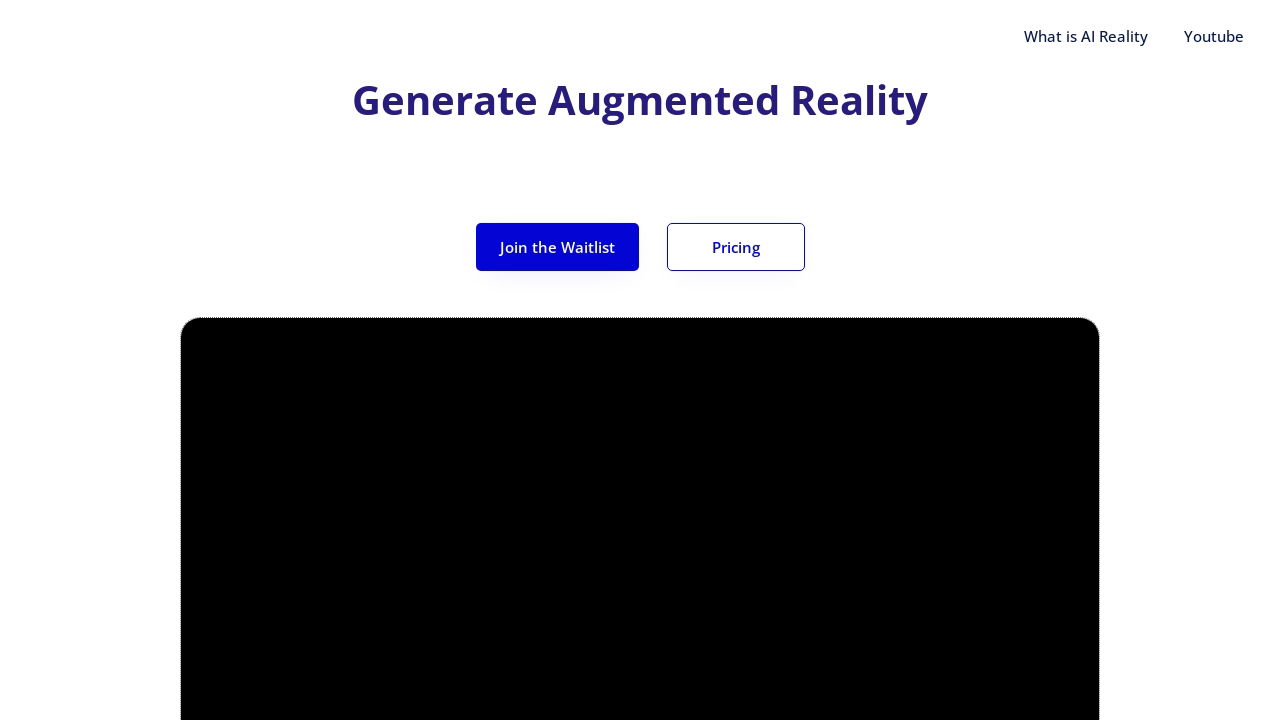
The lab employs robust security measures to protect sensitive data, including encryption, access controls, and regular security audits. Data privacy is paramount, and the lab adheres to all relevant regulations and ethical guidelines.
Data Annotation and Labeling
Accurate data annotation and labeling are crucial for training effective AI models. The lab employs a combination of automated and manual annotation techniques, ensuring high-quality labels for diverse data types.
Data Types and Annotation Methods
Data Type | Annotation Method | Example | Challenges |
---|---|---|---|
Images | Bounding boxes, semantic segmentation, polygon annotation | Object detection in images | High cost and time consumption |
Text | Named entity recognition, sentiment analysis, part-of-speech tagging | Analyzing customer reviews | Handling ambiguity and context |
Sensor Data | Time series analysis, anomaly detection | Monitoring equipment performance | Dealing with noisy and incomplete data |
Video | Action recognition, event detection, object tracking | Analyzing traffic flow | High computational cost |
Applications and Impact of Reality AI Lab Research
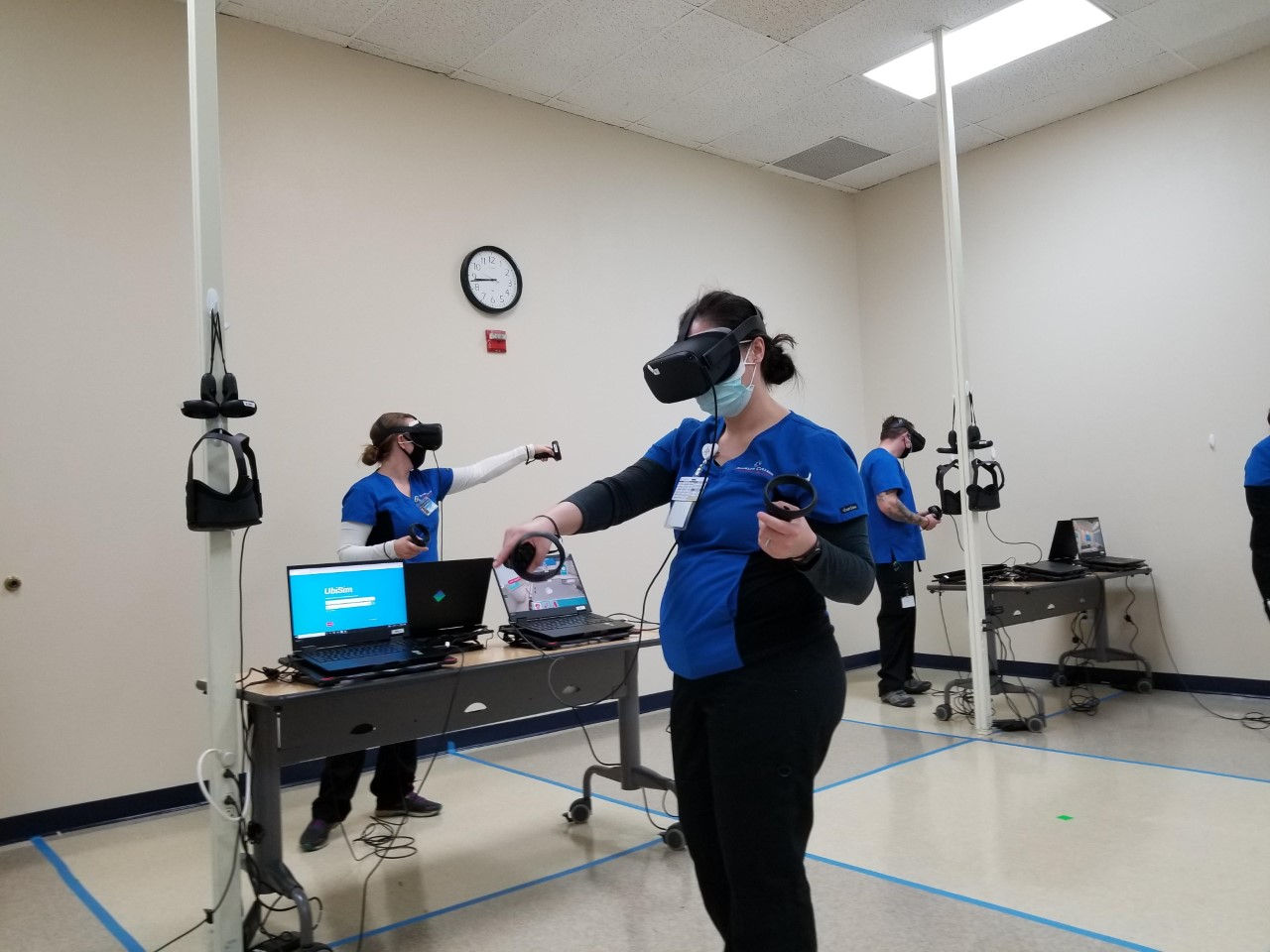
The Reality AI Lab’s research has the potential to significantly impact society and the economy.
Real-World Applications, Reality ai lab
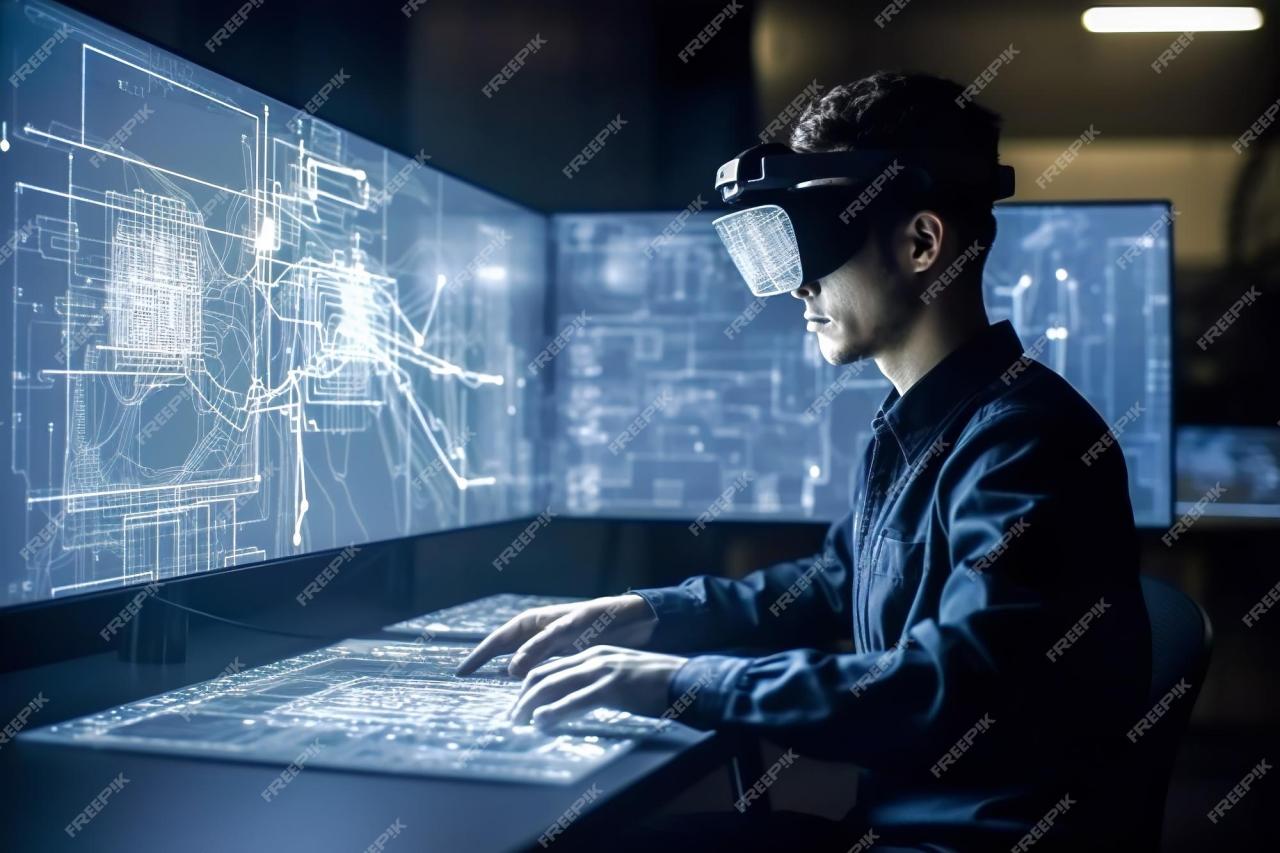
- Improved healthcare diagnostics and treatment.
- More efficient and sustainable resource management.
- Enhanced safety and security in urban environments.
- Increased productivity and efficiency in manufacturing.
- Development of more personalized and accessible services.
Societal Impact
Successful research projects can lead to improvements in public health, environmental protection, and social equity. AI-powered solutions can address critical societal challenges, leading to a more sustainable and equitable future.
Economic Implications
Advancements from the Reality AI Lab can drive economic growth by creating new industries, improving productivity, and fostering innovation. The development and deployment of AI technologies have the potential to create new job opportunities and boost economic competitiveness.
Challenges and Future Directions for Reality AI Lab
The Reality AI Lab faces several challenges, but also has exciting future directions for its research.
Challenges
Challenges include securing sufficient funding, navigating complex regulatory landscapes, and addressing ethical concerns surrounding AI development and deployment. Competition for talent and maintaining a competitive edge in the rapidly evolving field of AI are also significant challenges.
Future Directions
Long-term goals include developing more robust, explainable, and trustworthy AI systems. Focus areas include advancing research in areas like causal inference, federated learning, and AI safety. Exploring the potential of AI in tackling global challenges like climate change and healthcare disparities is a key priority.
Maintaining Competitive Edge
The Reality AI Lab will maintain its competitive edge by fostering collaboration with industry partners, attracting and retaining top talent, and investing in cutting-edge research infrastructure. A commitment to open science and knowledge sharing will also contribute to the lab’s continued success.
Visualizing Reality AI Lab’s Research
Visualizing the complex data and impacts of the Reality AI Lab’s research is crucial for communication and understanding.
Reality AI Lab focuses on pushing the boundaries of artificial intelligence, exploring its applications in various fields. If you’re wondering about the availability of other AI tools, you might check if chatgpt down? before diving into more complex AI projects. Understanding the limitations of current tech helps Reality AI Lab better refine its own research and development goals.
Data Visualization Tool
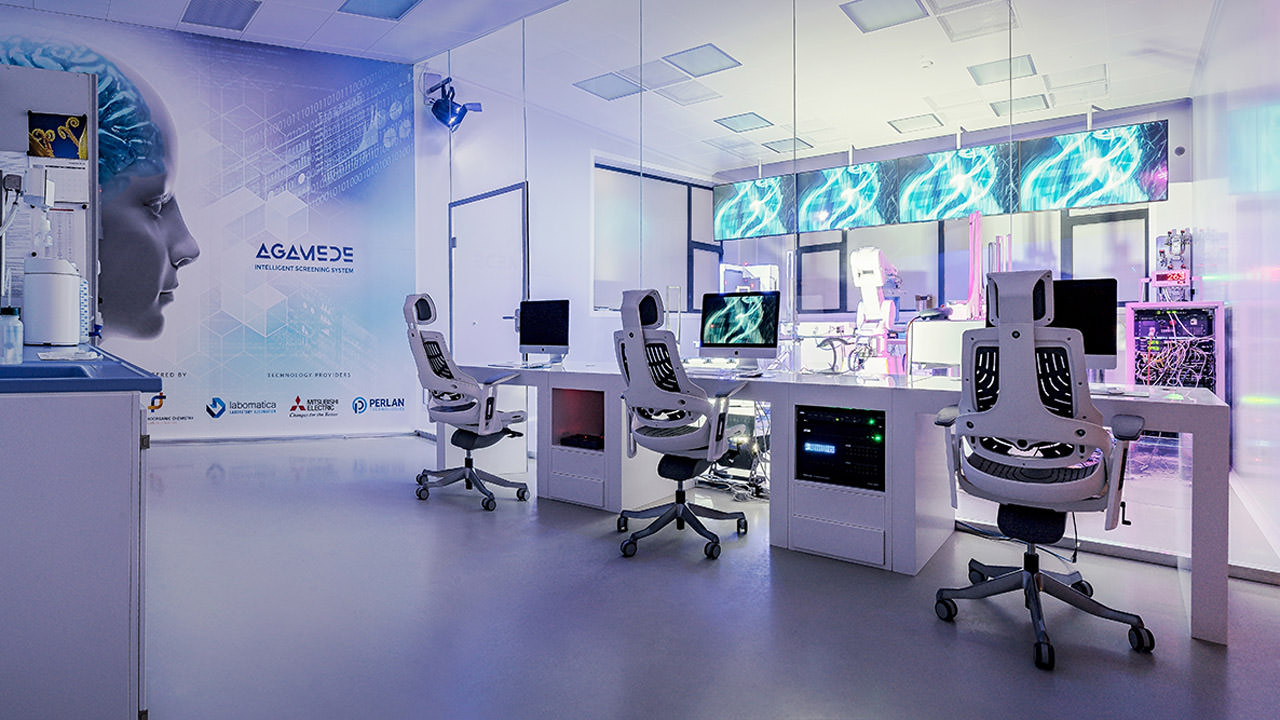
A visualization tool could employ interactive 3D graphs and network diagrams to illustrate the relationships between different data points and AI models. The tool would allow users to explore the complexity of data flows, model performance, and the impact of various parameters. Key information conveyed would include data volume, model accuracy, and the relationships between different data sources and AI models.
Color-coding and interactive elements would enhance the visualization’s clarity and user experience.
Visual Representation of Societal Impact
A compelling visual could use a before-and-after comparison to showcase the impact of AI-powered solutions on a specific societal issue, such as reducing traffic congestion in a city. The visualization would present data on traffic flow, travel times, and emissions before and after the implementation of an AI-powered traffic management system. This would effectively communicate the positive impact of the research on the city’s environment and quality of life.
A clear and concise legend would ensure easy interpretation of the data presented.
Reality AI Lab focuses on pushing the boundaries of AI in real-world applications. Think about how AI could improve drone operations, like those showcased at the awesome drone paris event. Seeing the latest drone tech there really helps us at Reality AI Lab understand the current challenges and opportunities for developing more robust and intelligent AI systems for aerial robotics.
Final Wrap-Up
Reality AI Lab represents a significant step forward in harnessing the power of AI for good. By focusing on real-world applications and prioritizing ethical considerations, we aim to create a future where AI technology is both beneficial and accessible to all. The challenges are substantial, but the potential rewards—a more efficient, equitable, and sustainable world—make the pursuit well worth the effort.
We invite you to explore our research and join us in shaping the future of AI.
Answers to Common Questions
What types of AI models does Reality AI Lab use?
We utilize a range of models, including deep learning networks (like CNNs and RNNs), reinforcement learning algorithms, and more, selecting the best approach for each specific application.
How does the lab address data privacy concerns?
Data privacy is paramount. We employ robust encryption, anonymization techniques, and adhere to strict data governance protocols to ensure the responsible handling of all data.
What is the lab’s funding model?
Funding is secured through a combination of grants, private investment, and collaborations with industry partners.
Who are the researchers at Reality AI Lab?
Our team comprises leading experts in AI, data science, ethics, and related fields from diverse backgrounds and institutions.